Meaningful low-dimensional representations of dynamical processes are essential to better understand the mechanisms underlying complex systems, from music composition to learning in both biological and artificial intelligence.
We suggest describing time-varying systems by considering the evolution of their geometrical and topological properties in time, by using a method based on persistent homology. In the static case, persistent homology allows one to provide a representation of a manifold paired with a continuous function as a collection of multisets of points and lines called persistence diagrams. The idea is to fingerprint the change of a variable-geometry space as a time series of persistence diagrams, and afterward compare such time series by using dynamic time warping.
As an application, we express some music features and their time dependency by updating the values of a function defined on a polyhedral surface, called the Tonnetz. Thereafter, we use this time-based representation to automatically classify three collections of compositions according to their style, and discuss the optimal time-granularity for the analysis of different musical genres.
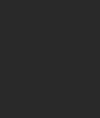